In today’s upgrading world, the ability to analyze and understand of data analytics is nessasry for businesses and researchers alike. This article writers various tools for data analysis, covering everything from data mapping to qualitative analysis. We’ll discuss the best tools for data analytics, visit their applications, and provide examples to help you choose the right tools for your needs.
Table of Contents
1. Introduction
2. Tools for Data Mapping
3. Best Tools for Data Analytics
4. Applications of Data Analytics Tools
5. Examples of Data Analysis Tools in Action
6. Tools for Qualitative Data Analysis
7. Conclusion
Introduction
Data analysis tools are essential for making sense of the enough amounts of information producing in our digital age. These tools help us organize, exploring, and analysis data, leading to better decision-making and insights. Whether you’re a business analyst, researcher, or data scientist, having the right tools at your disposal can significantly enhance your ability to extract valuable information from stright data.
Tools for Data Mapping / Data Analytics
The process of creating a relationship between different data models is called as Data mapping. It’s a sensitive step in data analytics, migration, and transfer of data form one to another place. Here are some popular tools for data mapping:
1. Talend Data Mapper: Part of Talend’s suite of data integration tools, it offers a visible interface for mapping difficult data structures.
2. Informatica PowerCenter: Known for its robust data mapping capabilities, especially for large-scale enterprise data integration projects.
3. Microsoft SQL Server Integration Services (SSIS): Offers data mapping features as part of its ETL (Extract, Transform, Load) capabilities.
4. Altova MapForce: A perfect data mapping tool that supports different data formats and can generate code for data transformations.
5. FME (Feature Manipulation Engine): Specializes in sensitive data transformation but can handle various data types.
These tools allow you to visually define relationships between different kinds of data fields, transformations, and ensure data consistency across systems.
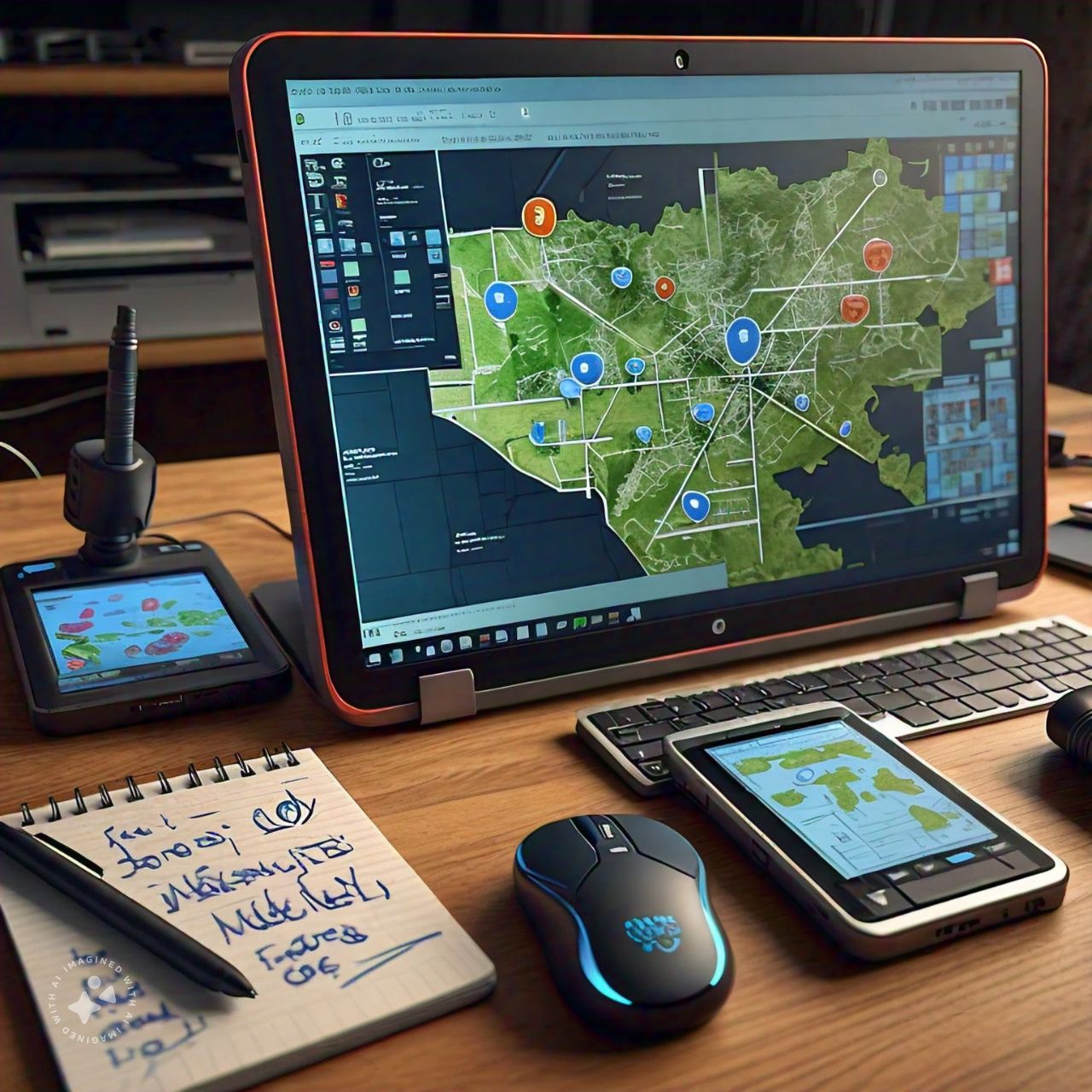
Best Tools for Data Analytics
Data analytics tools help you process, analyze, and visualize data to uncover insights. There are some of the best tools
1. Tableau: Known for its powerful data collecting and analysis capabilities and user-friendly interface.
2. Power BI: Microsoft’s business analytics tool, offering active visualizations and business intelligence features.
3. Python: with libraries like Pandas, NumPy, and Matplotlib: Ideal for data manipulation and analysis, with strong analysis capabilities.
4. R: A programming language specifically designed for statistical computing and graphics.
5. Statistical Analysis System (SAS): A comprehensive suite of advanced analytics tools, popular in large scale enterprises and academic research.
6. Google Analytics: Specifically for web analytics, it provides insights into website traffic and user behavior.
7. IBM SPSS: Offers advanced statistical analysis tools, popular in social sciences and market research.
These tools range from user-friendly interfaces for creating quick visualizations to powerful programming environments for complex statistical analyses.
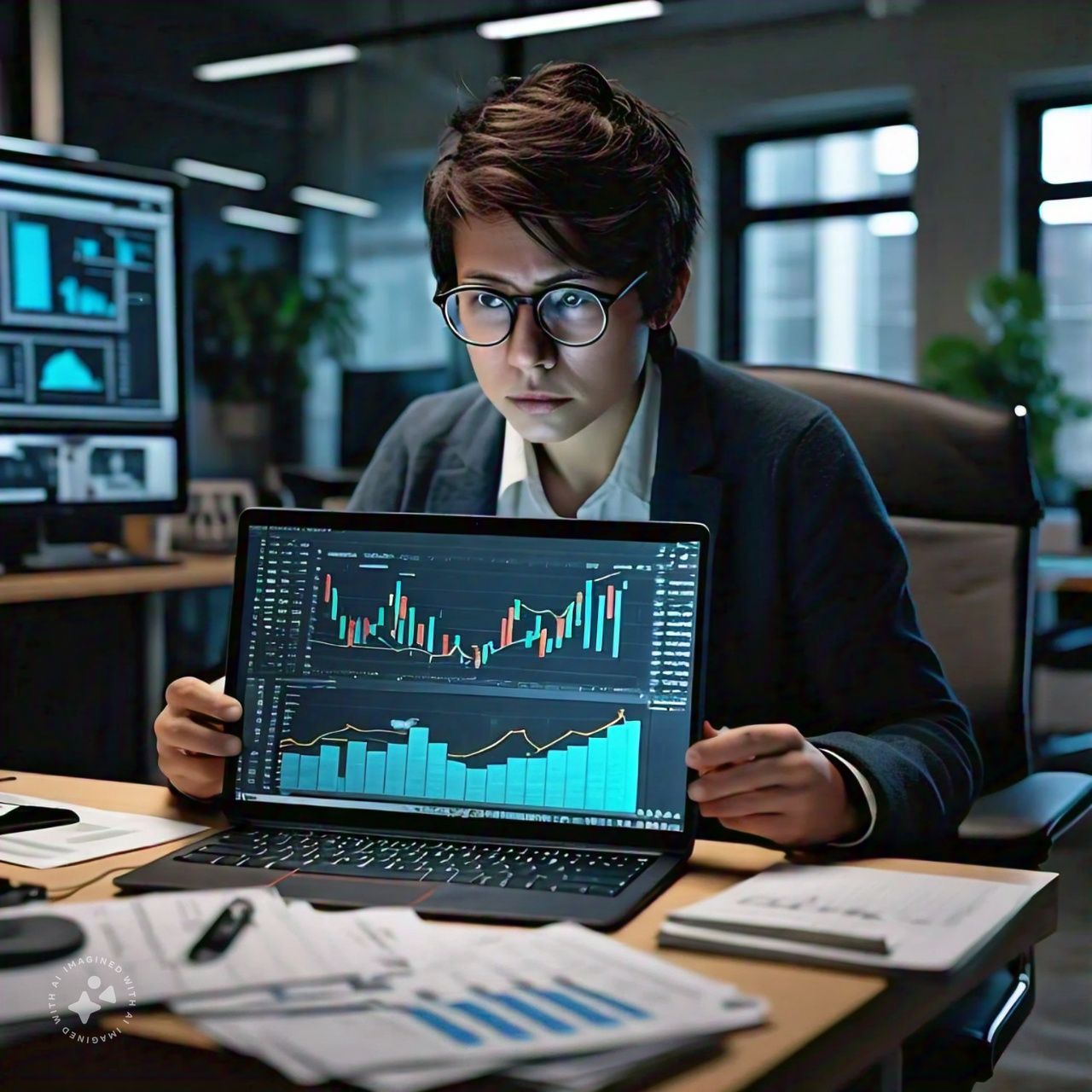
3.Applications of Data Analytics Tools
Data analytics are useful tools for a wide range of applications, including:
1. Business Intelligence: Analyzing sales data, customer behavior, and market trends to inform business strategies.
2. Financial Analysis: Forecasting, risk assessment, and fraud detection in the financial sector.
3. Healthcare: Analyzing patient data for better diagnoses, treatment plans, and public health monitoring.
4. Marketing: Understanding customer preferences, segmentation, and campaign performance analysis.
5. Supply Chain Management: Optimizing inventory levels, predicting demand, and improving logistics.
6. Scientific Research: Analyzing experimental data, testing hypotheses, and discovering patterns in large datasets.
7. Social Media Analysis: Tracking trends, sentiment analysis, and measuring engagement.
8. Internet of Things (IoT): Analyzing data from connected devices for predictive maintenance and process optimization.
The versatility of data analytics tools makes them indispensable across various industries and research fields.
Examples of Data Analysis Tools in Action
Let’s look at some practical examples of how these tools are used:
1. Tableau for Sales Analysis: A retail company uses Tableau to create interactive dashboards showing sales trends across different regions and product categories. This helps them identify top-performing products and areas needing improvement.
2. Python for Predictive Maintenance: A manufacturing company uses Python with sci-kit-learn to analyze sensor data from their machines. They build models to predict when equipment is likely to fail, allowing for proactive maintenance.
3. R for Medical Research: Researchers use R to analyze clinical trial data, performing statistical tests to determine the efficacy of new treatments.
4. Power BI for Marketing Analytics: A digital marketing agency uses Power BI to create reports that combine data from various sources like Google Analytics, social media platforms, and CRM systems. This gives their clients a comprehensive view of their marketing performance.
5. SAS for Fraud Detection: A bank uses SAS to analyze transaction patterns and flag potentially fraudulent activities in real-time.
These examples demonstrate how data analysis tools can be applied to solve real-world problems across different industries.
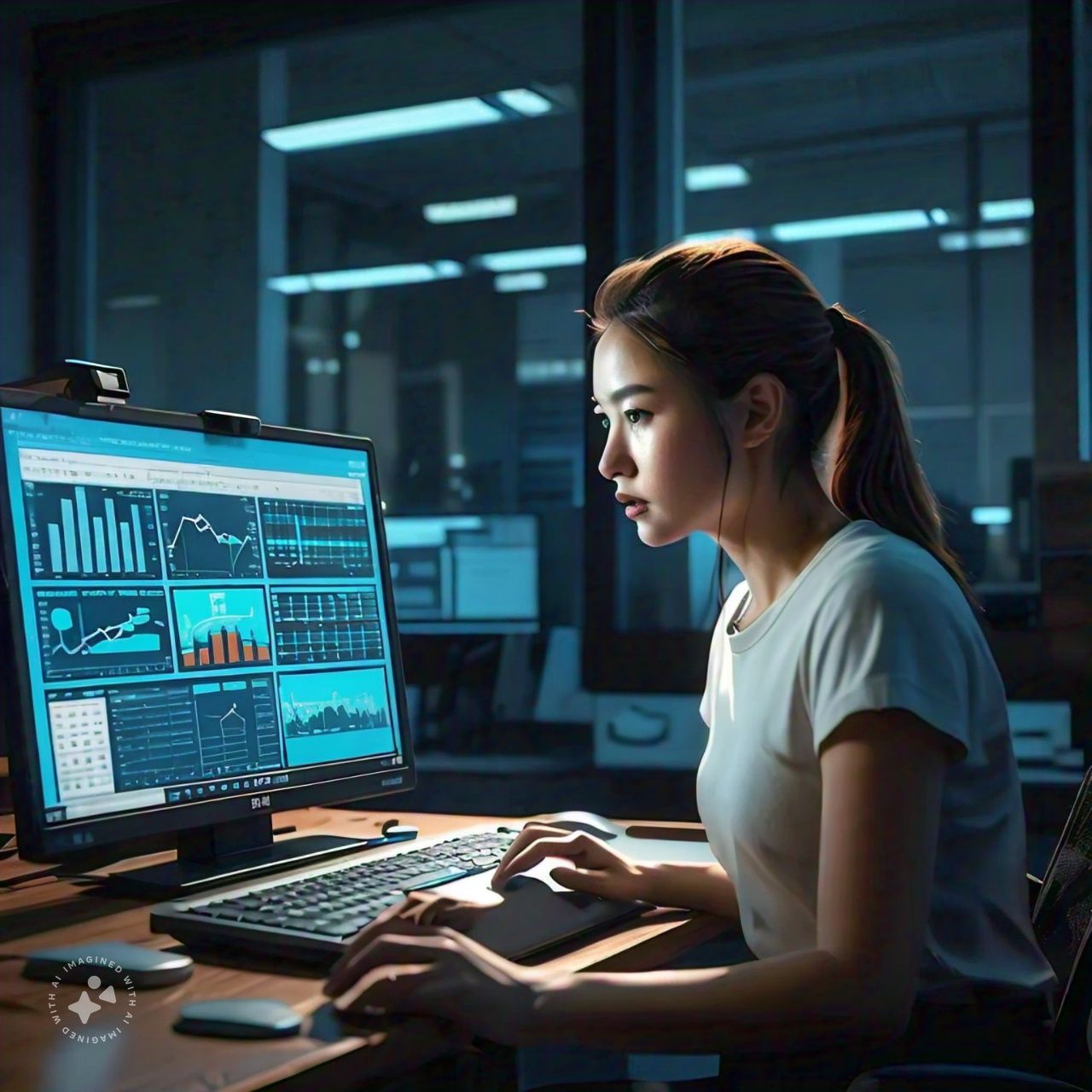
Tools for Qualitative Data Analysis or Analytics
While many data analysis tools focus on quantitative data, there are also specialized tools for analyzing qualitative data, such as text from interviews, open-ended survey responses, or social media posts. Here are some popular tools in this category:
1. NVivo: Helps researchers organize and analyze unstructured data like interviews, open-ended survey responses, articles, and social media content.
2. ATLAS.ti: Offers powerful tools for qualitative analysis of textual, graphical, audio, and video data.
3. MAXQDA: Supports mixed methods research, allowing analysis of both qualitative and quantitative data.
4. QDA Miner: Provides tools for coding, annotating, retrieving, and analyzing small and large collections of documents and images.
5. Quirkos: Offers a visual and intuitive interface for coding and analyzing qualitative data.
These tools help researchers identify themes, code data, and uncover insights from unstructured information, complementing the quantitative analysis tools we discussed earlier.
Conclusion
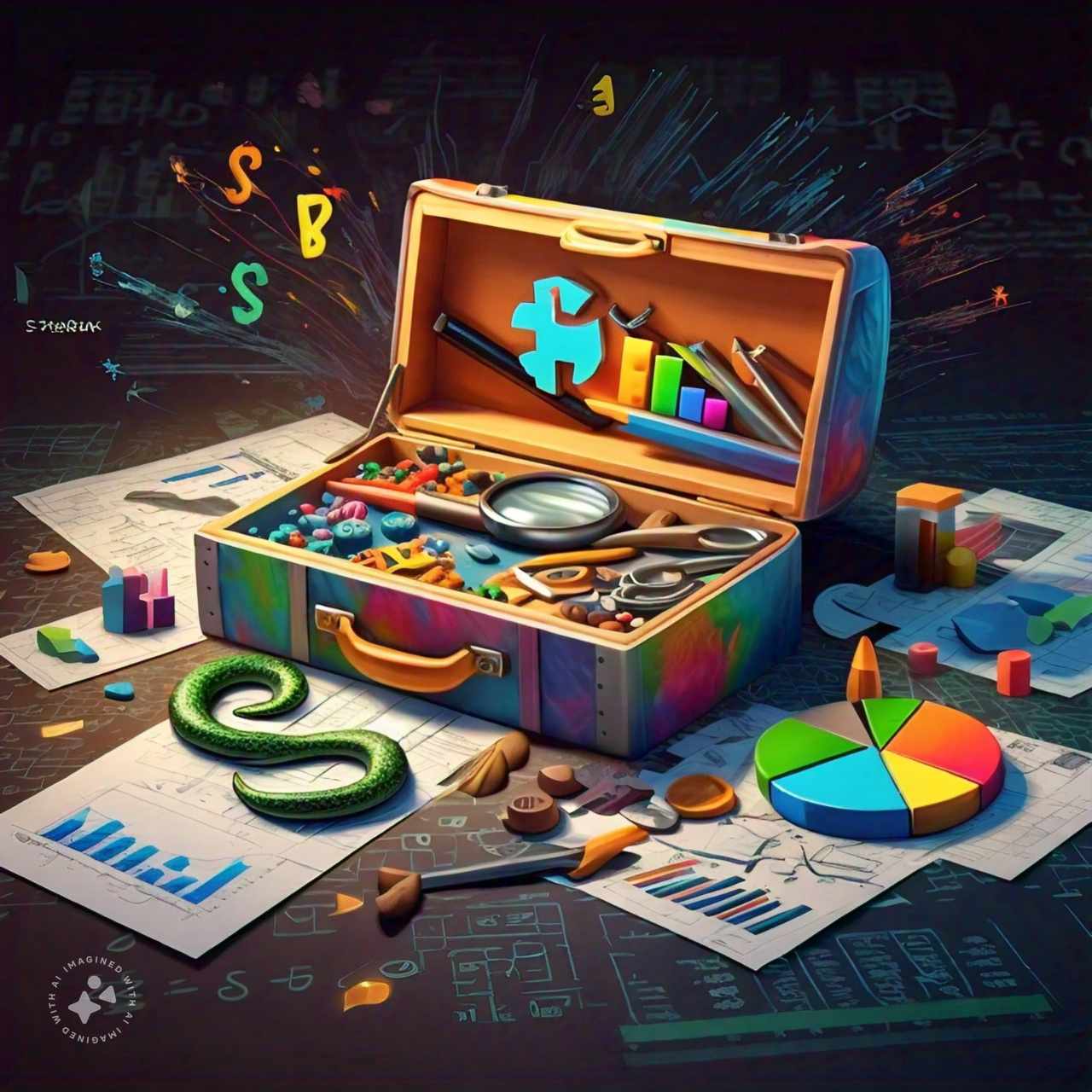
Excel is a tool many people know. It’s good for working with numbers in rows and columns. You can make charts and do math with Excel.
Python is computer language. It’s very good at working with all kinds of information. You can use it to clean up messy data. You can also use it to find patterns in your data. Python is free, but it takes time to learn.
R is another computer language. It’s like Python but made just for working with data. Many scientists and math people use R. It’s very good at doing hard math problems.
Tableau helps you make pretty pictures with your data. You can make charts that move and change. This helps people understand your information better. Tableau costs money, but it’s not too hard to learn.
Power BI is like Tableau. It helps you show your data nice ways. It works well with other Microsoft tools. If you use things like Excel a lot, Power BI might be good for you.
SAS is a tool for big companies. It can work with lots of data fast. It’s good at finding hidden patterns in data. SAS costs a lot money, but it’s very powerful.
Hadoop helps you work with very, very big amounts of data. It spreads the work over many computers. This makes it faster. Hadoop is free, but it’s hard to set up and use.
Spark is like Hadoop but newer. It can work with data very fast. It’s good for learning about data as it comes in. Spark is free too, but it’s also hard to learn.
SQL helps you work with data in big tables. It’s good at finding the exact information you need. Many other tools use SQL too. It’s not too hard to learn and is very useful.
Mechine Learning tools
There are also tools that help you guess what might happen. These are called machine learning tools. TensorFlow and scikit-learn are two of these. They can look at your data and find patterns. Then they can use these patterns to make guesses about new data.
Some tools help you work with words and language. NLTK and spaCy are two of these. They can help you understand what people are saying in text. This is good for looking at things like social media posts.
There are tools for special kinds of work too. If you work with money, you might use tools like Bloomberg Terminal or Eikon. These give you lots of information about money and markets.
If you work with maps and places, you might use tools like ArcGIS or QGIS. These help you make maps and look at data about different places.
As you can see, there are many, many tools to choose from. It’s okay to start small. Maybe begin with Excel or Google Sheets. These are easy to use and can do a lot. As you learn more, you can try bigger tools.
Remember, the best tool is the one that helps you do your job. It doesn’t have to be the fanciest or most expensive. It just needs to work for you.
When you’re learning new tool, take your time. It’s okay to make mistakes. That’s how you learn. Look for videos or classes that can help you. Many tools have groups of people who use them. These groups can be very helpful when you have questions.
Don’t forget that tools updating. New ones come out all the time. Old ones get better. It’s good to keep learning about new tools. But don’t feel like you need to know everything. Focus on what helps you most.
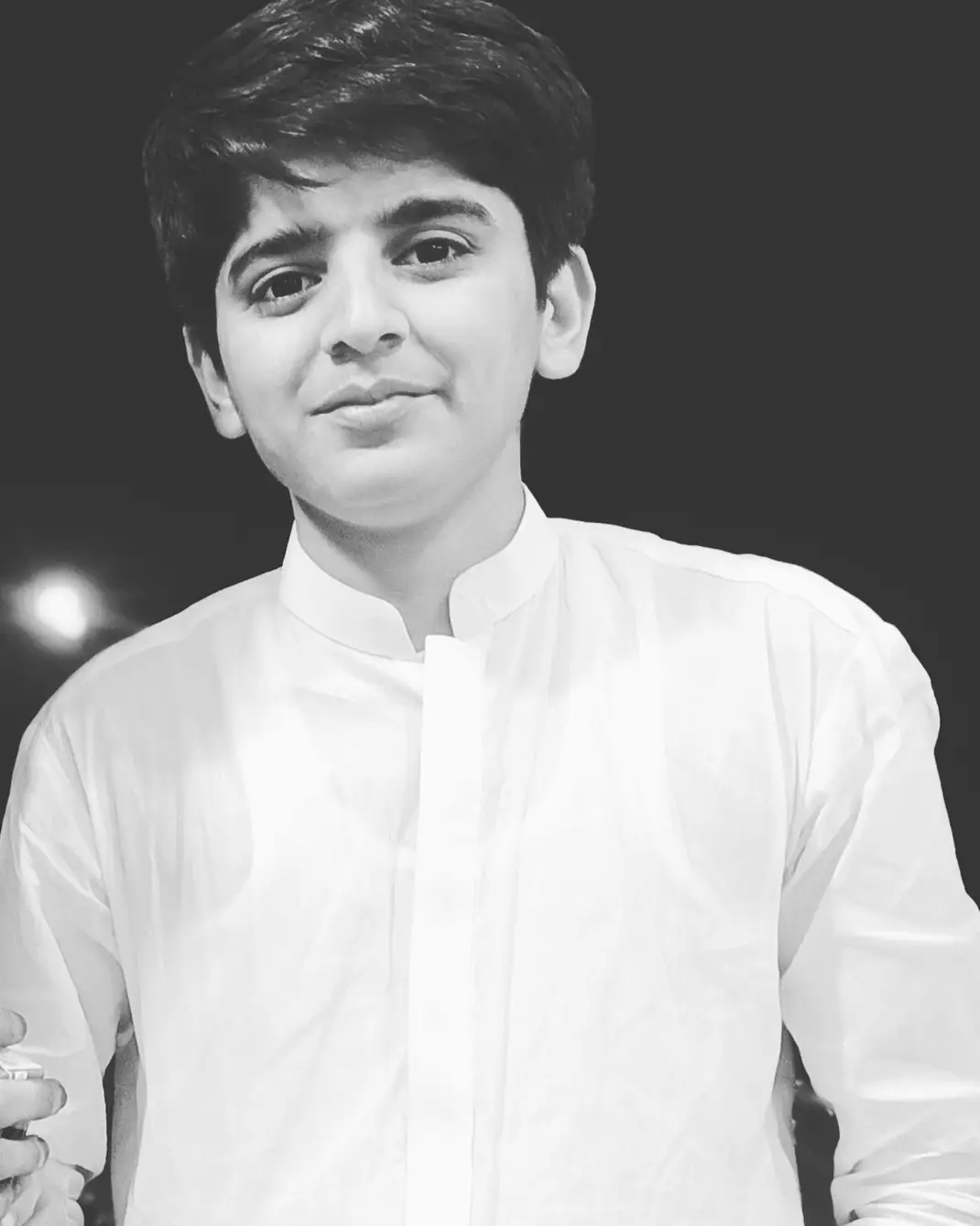
بِسْمِ ٱللَّٰهِ ٱلرَّحْمَٰنِ ٱلرَّحِيمِ
I’m Shafeeq Bugti I write about Business Softwares and created this blog in 2024. I Started using WordPress in 2024 and Embarked on a highly successful career as an entrepreneur in SaaS Community. I’m 23 year old entrepreneur. I live in Sui Dera Bugti, Balochistan, Pakistan